The innovative technique asks AI to find objects hidden from view by looking into the shadows, leading to safer autonomous vehicles, better-performing AR or faster warehouse automation.
When road collisions happen ahead of human drivers, they rely on signals such as brake or hazard lights from the vehcile/s ahead to be forewarned of the danger. Even current autonomous driving technologies only take note of the speed differential of the car ahead – performing emergency braking only a fraction of a second earlier, and safer, than a human.
Now new research by experts at MIT and Meta poses the question, if an autonomous vehicle could see around the car/s ahead, could it apply the brakes even sooner? If so, the ability could lead to softer autonomous braking and, in turn, fewer collisions, fewer injuries and improved traffic flow.
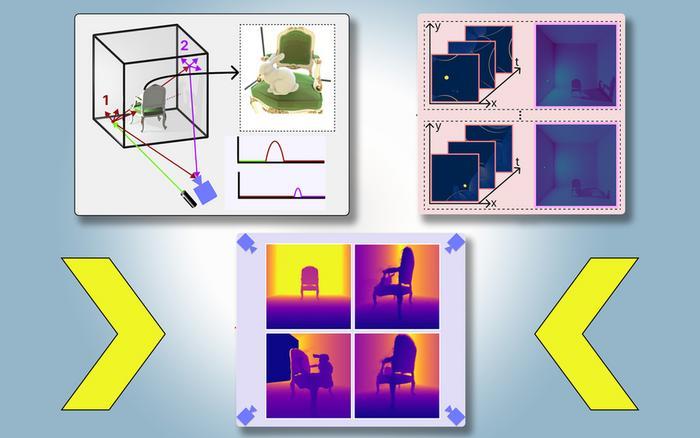
The example above shows the system modelling a rabbit in the chair, which is blocked from visible view. Image: Tzofi Klinghoffer, Ramesh Raskar, et al.
The computer vision technique developed and introduced by MIT graduate Tzofi Klinghoffer and others from MIT and Meta is called Plato-NeRF – named after Plato’s allegory of the cave in a passage from the Greek philosopher’s ‘Republic’, in which prisoners chained in a cave discern the reality of the outside world based on shadows cast on the cave wall.
Looking in the shadows
The method uses shadows to determine what lies in the areas blocked from view, creating a physically accurate 3D model of an entire scene with images from a single camera position. By combining lidar with machine learning, Plato-NeRF’s 3D reconstructions beat some existing AI techniques for accuracy, while also offering smoother reconstructed scenes in environments where the shadows are hard to see, such as those with high ambient light or dark backgrounds.
“Our key idea was to take these two things from different disciplines – multi-bounce lidar and machine learning – and pull them together,” said Klinghoffer. “And it turns out that when you bring them together, you find a lot of new opportunities to explore and get the best of both worlds.”
Differences between Plato-NeRF and existing software
While some existing machine learning approaches use generative AI to guess the presence of objects in unseen areas, these models can hallucinate, seeing objects that aren’t there. Other approaches that do use shadows to infer the shapes of hidden objects, meanwhile, do so with a colour image – which can struggle when shadows are hard to see.
Single-photon lidar illuminates a target point in the scene before reading the light that bounces back to the sensor. As most of the light scatters and bounces off other objects before returning to the sensor, however, it is hard to get definitive data. Plato-NeRF instead relies on these second bounces of light.
By tracking these secondary rays – those bouncing off the target point and heading to other points – Plato-NeRF can determine which points lie in shadow and, based on the location of the shadows, infer the geometry of hidden objects. “Every time we illuminate a point in the scene, we create new shadows,” said Klinghoffer. “Because we have these different illumination sources, we have a lot of light rays shooting around, so we carve out the region that is occluded and beyond the visible eye.”